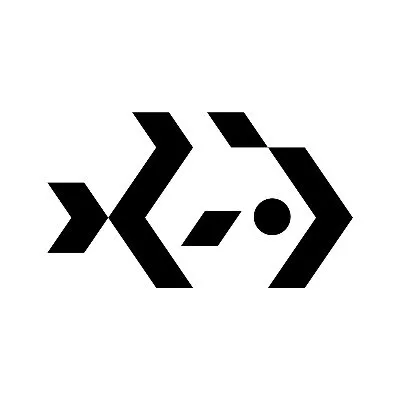
Bacalhau
Distributed Compute Over Data
Description
Bacalhau seeks to address deep rooted gaps in managing big data by giving developers low cost, decentralized tools that unlock a new collaborative ecosystem. It is a network of open compute resources made available to serve any data processing workload. Bacalhau enables users to run arbitrary docker containers and wasm images against data sto...
Additional Details
EXAMPLE USE CASES
LOG PROCESSING
Sanitize and process application logs at source before centralizing, resulting in reduced transport costs, quicker insights, and strict data privacy compliance.
EDGE ML TRAINING
Distributed ML training across remote devices without central data aggregation, leading to enhanced security, lower latency, and maintained model accuracy.
DISTRIBUTED DATA WAREHOUSE DATA
Virtual data warehouse with federated queries across distributed sources, enabling faster insights, cost savings, and access to real-time data.
DISTRIBUTED FLEET MANAGEMENT
Query device fleets instantly using Bacalhau’s OSQuery with a SQL-like engine, all without data centralization. Container support to swiftly audit, configure, and monitor health. Benefit from enhanced uptime, faster issue resolution, and reduced engineering effort.
PROCESSING OVER GEOGRAPHICALLY
Distributed Files Data processing across distributed storage and varied regions, resulting in significant cost savings, faster data processing, and minimized compliance risks.
RUNNING JOBS OVER UNRELIABLE NETWORKS
Decentralized job coordination for resilient execution across unstable networks, ensuring reliable job execution, fewer failures, and support for geo-distributed queues.
FEDERATED LEARNING WITH ISOLATED DIVISIONS
Jointly train ML models without sharing raw data across divisions. Enjoy superior model accuracy and minimized regulatory risks.
SHARED MACHINES
Coordinate experiments across shared computing resources without transferring data. Benefit from optimal hardware usage, accelerated research, and uncompromised data security.